“If at first you don’t succeed, try, try again.” Oh that’s nice, but really? How long should you keep trying…forever? What, if anything, should you do differently on your next try to improve chances of success?
What if I told you that some math geniuses at Northwestern University figured out a formula to determine whether an individual or group would eventually succeed or fail at something? While the model can't pinpoint the exact number of tries, the researchers did uncover the factors that empirically determine eventual success or terminal failure.
It sounds almost impossible, but the researchers published an eye-opening paper in the prestigious journal Nature that describes such a model.[i] To prove their point to statistical significance, the researchers needed multiple large datasets of individuals or groups who made consecutive attempts at an objective that only sometimes led to success.
In addition, the datasets needed to include some measure of improvement between failures and a clear definition of success. Finally, they wanted datasets from highly disparate domains to see if the models could be applied more broadly.
The three datasets they chose were:
NIH grant seekers, specifically those vying for the competitive R01 grants. Their measure of improvement was a percentile score the applicant received when the grant was denied, and success was defined as an application that resulted in the grant being awarded.
Entrepreneurs who founded multiple ventures over time. Improvement was measured by total funds raised during each venture and success was defined as either going public or selling the company at a high value within five years of the initial investment.
Terrorist Organizations. Yes, as insane as this sounds, the original paper did not make any apologies or note any ethical considerations about selecting this controversial dataset. Worse, the measure of improvement for the terrorist groups was how many people were wounded in subsequent attacks, and success was defined as killing at least one person in an attack. Of course, terrorist organizations are horrible, however, if we can learn from their terrible actions, we should.
It would be hard to argue that these aren’t very disparate datasets, which makes the results even more compelling.
When the researchers boiled down all the advanced math and data analysis, they found two determinants of success across all three datasets.
The amount of time between attempts. If the time between attempts became shorter with each try, the likelihood of success increased. If there was no temporal pattern observed between attempts, the model more likely predicted ultimate failure. It would seem that “fail fast” is not a corporate mantra for nothing.
The amount of improvement between attempts. Whether it be the NIH grant percentile score, the total funding for the next venture, or the number of people wounded in the next terrorist attack, substantial improvement between attempts was indicative of ultimate success. Unsuccessful groups showed no statistically significant performance gains between attempts in any of the domains.
Tying together the timing-based or temporal variable and the variable measuring improvement, the researchers came up with a magical constant k, which as they describe “measure(s) the number of previous attempts one considers when formulating a new one.” So for example, in the NIH grant application case, the more parts of previous grant applications researchers can use (things they got right), the more they’re likely to succeed.
Essentially, k is a measure of how much learning and feedback people put into their next attempt. The fascinating thing is when you plot out values for k, there is a clear area that the researchers called the stagnation region, which represents individuals or teams who “reject prior attempts and thrash around for new versions, not gaining enough feedback to initiate a pattern of intelligent improvements.”
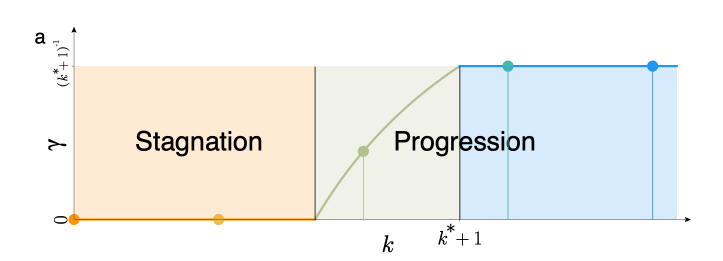
The Northwestern paper predicting success and failure is both simple and profound. When repeatedly trying to succeed at something and running into failure, you should: 1) carefully consider all feedback and data from your failure, 2) salvage as much of the “good” aspects of your attempt on your subsequent one, and 3) continue to both improve the speed of each attempt as well as watch for measurable improvements on each iteration.
If you don’t see consistent measurable improvement on subsequent attempts, or your timing between attempts does not get shorter, you’re probably not incorporating enough of the feedback from previous attempts.
The book Outsmart the Learning Curve will bring this research to life with the wrenching story of a single data point in the study—a promising medical researcher who failed on his first seven attempts to get NIH R01 grant funding. You’ll learn why he initially failed, how he kept going in the face of all this failure, and the process he followed to eventually succeed.
[i] Yin, Yian, Yang Wang, James A. Evans, and Dashun Wang. “Quantifying the Dynamics of Failure Across Science, Startups and Security.” Nature 575, no. 7781 (October 30, 2019): 190–94. https://doi.org/10.1038/s41586-019-1725-y.